A conversation with Connor Zwick, CEO & Co-founder of Speak.
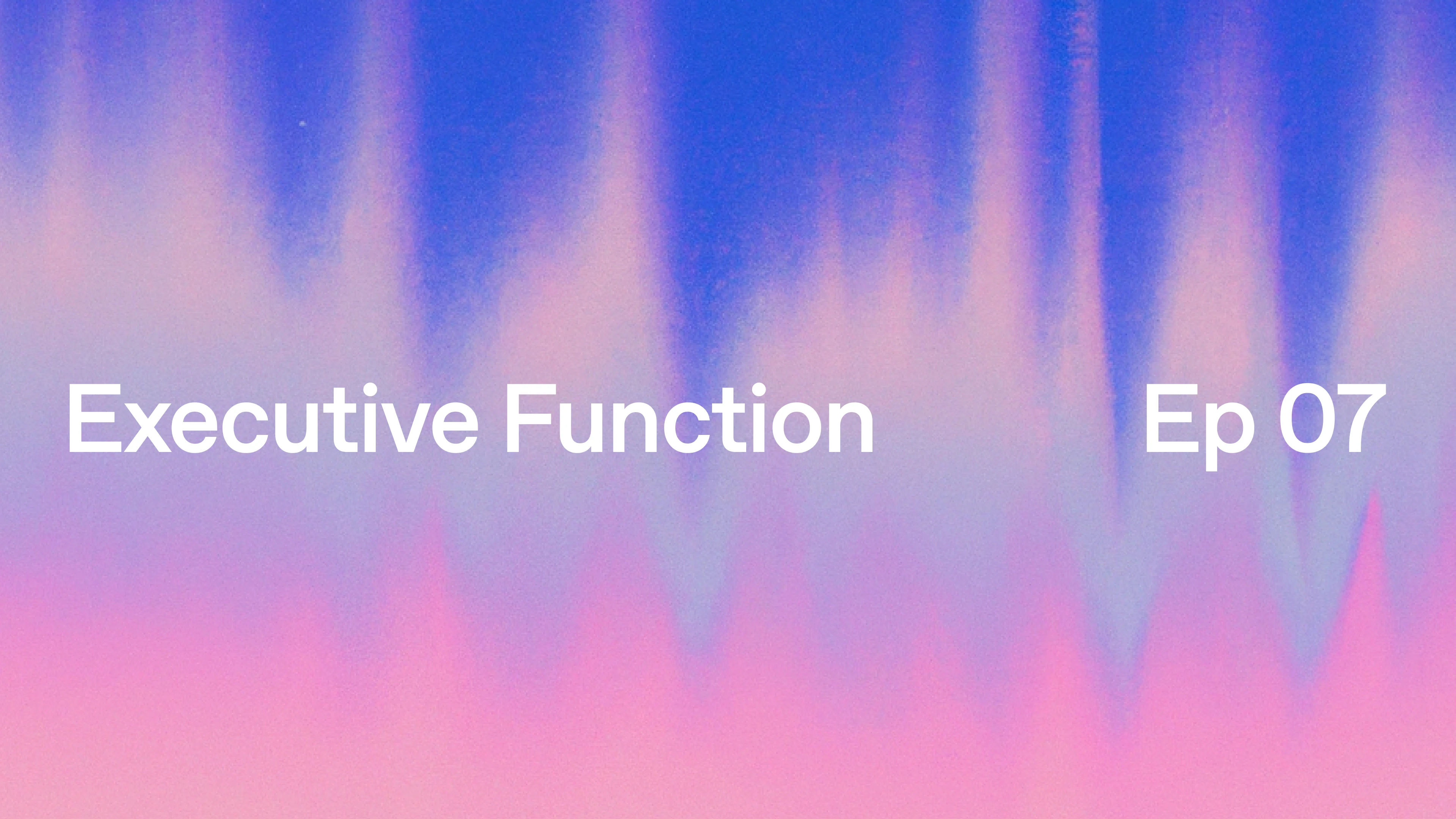
Our Executive Function series features perspectives from leaders driving transformation through AI.
Speak(opens in a new window) is a language learning app that sets users on the path to fluency with the world’s most advanced AI tutor. We spoke with Connor Zwick, CEO of Speak, about how AI is reshaping language learning, the breakthroughs enabling more natural AI tutors, and the challenges of scaling an AI startup in a rapidly evolving technical landscape.
I think if I look back over the last 10+ years, there are a bunch of different moments that come to mind—things that really left an impression on me and changed the way I think about AI.
Obviously, in 2012, there was the AlexNet paper, and even just doing image recognition with these deep neural networks was really, really cool. Then AlphaGo was another big moment. But for me personally, I was up close and personal with AI in 2015. My co-founder and I were doing our own independent AI research, trying to learn as much as possible—reading all the papers, implementing things. We scraped a bunch of YouTube data as a side project.
We put all the data into the model, not really knowing what to expect. On the first training run, we came back a few hours later and tested it. We had built a model that was better than the state of the art in accent detection—classifying what accent someone was speaking with.
“We realized deep learning was going to be incredibly powerful. If you just had enough data, it could do amazing things and, in many cases, completely smash state of the art…”
For us, it was about how to integrate deep learning into the language learning experience. The first few years of Speak were focused on building really good speaking experiences. It was actually really obvious because, before us, language learning apps didn’t really have speaking components. If they did, they didn’t have models that could robustly understand someone speaking with an accent.
Speech recognition models were super inaccurate for accented speech. But because we were able to quickly build speech recognition that worked better than any of the big models at the time, we saw an opportunity to throw that into a basic product experience and already have something game-changing.
This might not be the answer everyone wants to hear, but I believe that if you want to be an AI product leader, you need to have a deep technical intuition for how the technology and models work. Without that, you won’t have a good sense of which problems will be solved in the next month or 12 months, versus problems that will take a long time to get right.
If you do have that intuition, you can build for the future. For example, we sometimes build things that are cost-prohibitive today, knowing that costs will go down in a year. Or we design around model weaknesses, knowing they will improve over time.
Understanding the difference between 90% accuracy, 98%, 99%, and 99.9%—and how that impacts the product experience—is crucial. The difference between 90% and 99.9% is a completely different ballgame, and being able to predict when that curve will go up is essential for making sound product decisions.
That’s easy—OpenAI’s real-time API and multimodality for audio. For our use case, where we’re building a superhuman AI speaking tutor that can help learners achieve fluency, having a rich understanding of what a learner is trying to say—beyond just transcribing their words—is critical. Instantly understanding tone, pronunciation, and intent, and then immediately responding with open-ended, natural feedback that matches the learner’s tone, is the holy grail of AI tutoring.
People talk about reasoning as the next frontier, and I agree. For us, the best human teachers stand out because they can design great learning plans and curricula, think deeply about student progress, and make adjustments accordingly. Having super-agentic reasoning capabilities in AI will be a huge breakthrough for language learning. It’s not the most obvious AI advancement for our space, but it will have a massive impact on making AI tutors as effective as the best human teachers.
There are billions of people trying to learn English and other languages, but there aren’t enough quality human teachers to meet that demand. Most people have had to rely on books or online videos, which aren’t the same as real conversations. At the end of the day, people learn languages to connect with other humans, not AI. Even when AI reaches superhuman levels, there will always be a need for real human practice.
“It’s not about replacing human teachers. It’s about making language tutoring better and more available to everyone around the globe.”
The most important thing is having the right people. A big cultural cornerstone for us is curiosity. We want people who are self-motivated and eager to explore how AI can scale their impact.
ChatGPT has this “blank canvas” problem where people don’t realize how they can use it until they randomly think of an application. AI is incredibly versatile, and we encourage our team to keep asking, “Could I be using AI for this?” and testing it out.
Everything can improve, but at this point, it’s about squeezing the juice out of the orange—building the best possible product using what’s available today. There are still huge technical challenges in applying AI effectively, and we call this our “ML scaffolding”—the technology that powers the entire product experience.
We’ve been at this for a while, so we have a head start, but there’s still a long way to go. Even if AI stopped advancing today, we have years’ worth of exciting work ahead.
“These models are particularly good at language, interacting with people, and using language. In many other industries there might still need to be some breakthroughs before there is truly transformative effects, I actually think we’ve got everything we need.”
Speak leverages OpenAI models to power its language learning curriculum across modalities such as audio and text, providing interactive speaking exercises, personalized tutors, and more.